Very short-term load forecaster based on a neural network technique for smart grid control
Palabras clave :
Smart grid
Energy demand
Very short-term forecaste
Fecha de publicación :
2020
Nota:
This article is an open access
article distributed under the terms and conditions of the Creative Commons Attribution
(CC BY) license (http://creativecommons.org/licenses/by/4.0/).
Cita:
Rodríguez, F. (Fermín); Martín, F. (Fernando); Fontán, L. (Luis); et al. "Very short-term load forecaster based on a neural network technique for smart grid control". Energies. 13 (19), 2020, 5210
Aparece en las colecciones:
Estadísticas e impacto
0 citas en

0 citas en
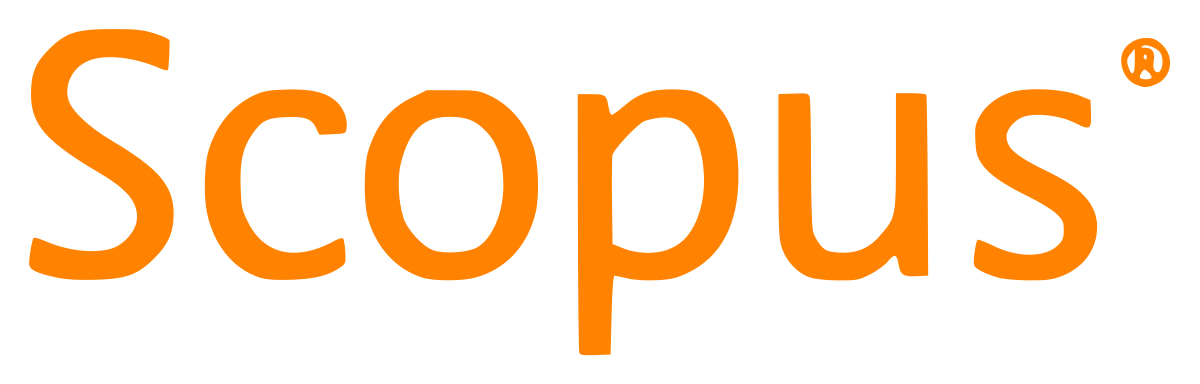
Los ítems de Dadun están protegidos por copyright, con todos los derechos reservados, a menos que se indique lo contrario.